Find what you need to succeed.
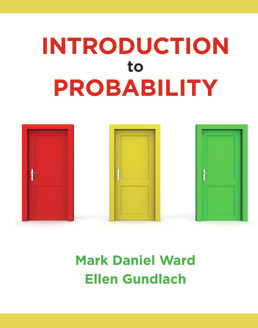
Introduction to Probability
First Edition ©2016 Mark Ward; Ellen Gundlach Formats: Digital & Print
As low as C$36.99
As low as C$36.99
Authors
-
Mark Ward
Mark Daniel Ward is an Associate Professor of Statistics at Purdue University. He has held visiting faculty positions at The George Washington University, the University of Maryland, the University of Paris 13, and a lecturer position at the University of Pennsylvania. He received his Ph.D. from Purdue University in Mathematics with Specialization in Computational Science (2005), M.S. in Applied Mathematics Science from the University of Wisconsin, Madison (2003), and B.S. in Mathematics and Computer Science from Denison University (1999). His research interests include probabilistic, combinatorial, and analytic techniques for the analysis of algorithms and data structures. Since 2008, he has been the Undergraduate Chair in Statistics at Purdue, and the Associate Director for Actuarial Science. Dr. Ward is currently the Principal Investigator for the NSF grant "MCTP: Sophomore Transitions: Bridges into a Statistics Major and Big Data Research Experiences via Learning Communities" (NSF-DMS #1246818, 2013-2018). He is also an Associate Director of the Center for Science of Information (NSF-CCF #0939370, 2010-2015).
-
Ellen Gundlach
Ellen Gundlach has been teaching introductory statistics and probability classes at Purdue University as a continuing lecturer since 2002, with prior experience teaching mathematics or chemistry classes at Purdue, Ivy Tech Community College of Indiana, The Ohio State University, and Florida State University.  She is an associate editor of CAUSEweb and editor of the MERLOT Statistics Board.  Her research interests include K12 outreach activities (ASA’s first Hands-on Statistics Activity grand prize winner in 2010), online and hybrid teaching (Indiana Council for Continuing Education’s Course of the Year award in 2011), T.A. training, academic misconduct, statistical literacy, and using social media in statistics courses. She enjoys spending time with her sons Philip and Callum, playing the flute with several local groups, and supporting (and formerly skating with) the Lafayette Brawlin’ Dolls roller derby team. 
Table of Contents
I Randomness
1 Outcomes, Events, and Sample Spaces
1.1 Introduction
1.2 Complements and DeMorgans Laws
1.3 Exercises
1.3.1 Practice
1.3.2 Extensions
1.3.3 Advanced
2 Probability
2.1 Introduction
2.2 Equally-Likely Events
2.3 Complementary Probabilities; Probabilities of Subsets
2.4 Inclusion-Exclusion
2.5 More Examples of Probabilities of Events
2.6 Exercises
2.6.1 Practice
2.6.2 Extensions
2.6.3 Advanced
3 Independent Events
3.1 Introduction
3.2 Some Nice Facts About Independence
3.3 Probability of Good Occurring Before Bad
3.4 Exercises
3.4.1 Practice
3.4.2 Extensions
3.4.3 Advanced
4 Conditional Probability
4.1 Introduction
4.2 Distributive Laws
4.3 Conditional Probabilities Satisfy the Standard Probability Axioms
4.4 Exercises
4.4.1 Practice
4.4.2 Extensions
4.4.3 Advanced
5 Bayes Theorem
5.1 Introduction to Versions of Bayes Theorem
5.2 Multiplication with Conditional Probabilities
5.3 Exercises
5.3.1 Practice
5.3.2 Extensions
5.3.3 Advanced
6 Review of Randomness
6.1 Summary of Randomness
6.2 Exercises
II Discrete Random Variables
7 Discrete Versus Continuous Random Variables
7.1 Introduction
7.2 Examples
7.3 Exercises
7.3.1 Practice
7.3.2 Extensions
7.3.3 Advanced
8 Probability Mass Functions and CDFs
8.1 Introduction
8.2 Examples
8.3 Properties of the Mass and CDF
8.4 More Examples
8.5 Exercises
8.5.1 Practice
8.5.2 Extensions
8.5.3 Advanced
9 Independence and Conditioning
9.1 Joint Probability Mass Functions
9.2 Independent Random Variables
9.3 Three or More Random Variables That Are Independent
9.4 Conditional Probability Mass Functions
9.5 Exercises
9.5.1 Practice
9.5.2 Extensions
9.5.3 Advanced
10 Expected Values of Discrete Random Variables
10.1 Introduction
10.2 Examples
10.3 Exercises
10.3.1 Practice
10.3.2 Extensions
11 Expected Values of Sums of Random Variables
11.1 Introduction
11.2 Examples
11.3 Exercises
11.3.1 Practice
11.3.2 Extensions
12 Variance of Discrete Random Variables
12.1 Introduction.
12.2 Variance
12.3 Five Friendly Facts with Independence
12.4 Exercises
12.4.1 Practice
12.4.2 Extensions
12.4.3 Advanced
13 Review of Discrete Random Variables
13.1 Summary of Discrete Random Variables
13.2 Exercises
III Named Discrete Random Variables
14 Bernoulli Random Variables
14.1 Introduction
14.2 Examples
14.3 Exercises
14.3.1 Practice
14.3.2 Extensions
14.3.3 Advanced
15 Binomial Random Variables
15.1 Introduction
15.2 Examples
15.3 Exercises
15.3.1 Practice
15.3.2 Extensions
15.3.3 Advanced
16 Geometric Random Variables
6.1 Introduction
16.2 Special Features of the Geometric Distribution
16.3 The Number of Failures
16.4 Geometric Memoryless Property
16.5 Random Variables That Are Not Geometric
16.6 Exercises
16.6.1 Practice
16.6.2 Extensions
16.6.3 Advanced
17 Negative Binomial Random Variables
17.1 Introduction
17.2 Examples
17.3 Exercises
17.3.1 Practice
17.3.2 Extensions
18 Poisson Random Variables
18.1 Introduction
18.2 Sums of Independent Poisson Random Variables
18.3 Using the Poisson as an Approximation to the Binomial
18.4 Exercises
18.4.1 Practice
18.4.2 Extensions
18.4.3 Advanced
19 Hypergeometric Random Variables
19.1 Introduction
19.2 Examples
19.3 Using the Binomial as an Approximation to the Hypergeometric
19.4 Exercises
19.4.1 Practice
19.4.2 Extensions
20 Discrete Uniform Random Variables
20.1 Introduction
20.2 Examples
20.3 Exercises
20.3.1 Practice
20.3.2 Extensions
20.3.3 Advanced
21 Review of Named Discrete Random Variables
21.1 Summing up: How do you tell all these random variables apart?
21.2 Exercises
21.3 Review Problems
IV Counting
22 Introduction to Counting
22.1 Introduction
22.2 Sampling With Versus Without Replacement; With Versus Without Regard to Order
22.3 Counting: Seating Arrangements
22.4 Exercises
22.4.1 Practice
22.4.2 Extensions
22.4.3 Seating Arrangement Problems
23 Two Case Studies in Counting
23.1 Poker Hands
23.1.1 Straight Flush
23.1.2 Four Of A Kind
23.1.3 Full House
23.1.4 Flush
23.1.5 Straight
23.1.6 Three Of A Kind
23.1.7 Two Pair
23.1.8 One Pair
23.2 Yahtzee
23.2.1 Upper Section
23.2.2 Three Of A Kind
23.2.3 Four Of A Kind
23.2.4 Full House
23.2.5 Small Straight
23.2.6 Large Straight
23.2.7 Yahtzee
V Continuous Random Variables
24 Continuous Random Variables and PDFs
24.1 Introduction
24.2 Examples
24.3 Exercises
24.3.1 Practice
24.3.2 Extensions
24.3.3 Advanced
25 Joint Densities
25.1 Introduction
25.2 Examples
25.3 Exercises
25.3.1 Practice
25.3.2 Extensions
25.3.3 Advanced
26 Independent Continuous Random Variables
26.1 Introduction
26.2 Examples
26.3 Exercises
26.3.1 Practice
26.3.2 Extensions
26.3.3 Advanced
27 Conditional Distributions
27.1 Introduction
27.2 Examples
27.3 Exercises
27.3.1 Practice
27.3.2 Extensions
28 Expected Values of Continuous Random Variables
28.1 Introduction
28.2 Some Generalizations about Expected Values
28.3 Some Applied Problems with Expected Values
28.4 Exercises
28.4.1 Practice
28.4.2 Extensions
28.4.3 Advanced
29 Variance of Continuous Random Variables
29.1 Variance of a Continuous Random Variable
29.2 Expected Values of Functions of One Continuous Random Variable
29.3 Expected Values of Functions of Two Continuous Random Variables
29.4 More Friendly Facts about Continuous Random Variables
29.5 Exercises
29.5.1 Practice
29.5.2 Extensions
29.5.3 Advanced
30 Review of Continuous Random Variables
30.1 Summary of Continuous Random Variables
30.2 Exercises
VI Named Continuous Random Variables
31 Continuous Uniform Random Variables
31.1 Introduction
31.2 Examples
31.3 Linear Scaling of a Uniform Random Variable
31.4 Exercises
31.4.1 Practice
31.4.2 Extensions
31.4.3 Advanced
32 Exponential Random Variables
32.1 Introduction
32.2 Average and Variance
32.3 Properties of Exponential Random Variables
32.3.1 Complement of the CDF
32.3.2 Memoryless Property of Exponential Random Variables
32.3.3 Minimum of Independent Exponential Random Variables
32.3.4 Poisson Process
32.3.5 Moments of an Exponential Random Variable (Optional)
32.4 Exercises .
32.4.1 Practice
32.4.2 Extensions
32.4.3 Advanced
33 Gamma Random Variables
33.1 Introduction
33.2 Examples
33.3 Exercises
33.3.1 Practice
33.3.2 Extensions
33.3.3 Advanced
34 Beta Random Variables
34.1 Introduction
34.2 Examples
34.3 Exercises
34.3.1 Practice
34.3.2 Extensions
35 Normal Random Variables
35.1 Introduction
35.2 Using the Normal Distribution: Scaling and Transforming to Standard Normal
35.3 \Backwards" Normal Problems
35.4 Summary: How to Distinguish a \Forward" Versus \Backwards" Normal Problem?
35.5 Exercises
35.5.1 Practice
35.5.2 Extensions
35.5.3 Advanced
36 Sums of Independent Normal Random Variables
36.1 The Sum of Independent Normal Random Variables is Normally Distributed
36.2 Why the Sum of Independent Normals is Normal Too (Optional)
36.3 Exercises
36.3.1 Practice
36.3.2 Extensions
36.3.3 Advanced
37 Central Limit Theorem
37.1 Introduction
37.2 Laws of Large Numbers
37.3 Central Limit Theorem
37.4 Applications of the Central Limit Theorem to Sums of Continuous Random Variables
37.5 Applications of the Central Limit Theorem to Sums of Discrete Random Variables
37.6 Normal Approximations to Binomial Random Variables
37.7 Normal Approximations to Poisson Random Variables
37.8 Exercises
37.8.1 Practice
37.8.2 Extensions
38 Review of Named Continuous Random Variables
38.1 Summing up: How do you tell all these random variables apart?
38.2 Exercises
VII Additional Topics
39 Variance of Sums; Covariance; Correlation
39.1 Introduction
39.2 Motivation for Covariance
39.3 Properties of the Covariance
39.4 Examples of Covariance
39.5 Linearity of the Covariance
39.6 Correlation
39.7 Exercises
39.7.1 Practice
39.7.2 Extensions
39.7.3 Advanced
40 Conditional Expectation
40.1 Introduction
40.2 Examples
40.3 Exercises
40.3.1 Practice
40.3.2 Extensions
40.3.3 Advanced.
41 Markov and Chebyshev Inequalities
41.1 Introduction
41.2 Markov Inequality
41.3 Chebyshev Inequality
41.4 Exercises
41.4.1 Practice
41.4.2 Extensions
42 Order Statistics
42.1 Introduction
42.2 Examples
42.3 Joint Density and Joint CDF of Order Statistics
42.4 Exercises
42.4.1 Practice
42.4.2 Extensions
43 Moment Generating Functions
43.1 A Brief Introduction to Generating Functions
43.2 Moment Generating Functions
43.3 Moment Generating Functions of Discrete Random Variables
43.4 Moment Generating Functions of Continuous Random Variables
43.5 Appendix: Building a Generating Function
43.6 Exercises
44 Transformations of One or Two Random Variables
44.1 Distribution of a Function of One Continuous Random Variable
44.2 Joint Density of Two Random Variables That Are Functions of Another Pair of Random Variables
44.3 Exercises
44.3.1 Practice
44.3.2 Extensions
44.3.3 Advanced
45 Review Questions for All Chapters
Product Updates
Authors
-
Mark Ward
Mark Daniel Ward is an Associate Professor of Statistics at Purdue University. He has held visiting faculty positions at The George Washington University, the University of Maryland, the University of Paris 13, and a lecturer position at the University of Pennsylvania. He received his Ph.D. from Purdue University in Mathematics with Specialization in Computational Science (2005), M.S. in Applied Mathematics Science from the University of Wisconsin, Madison (2003), and B.S. in Mathematics and Computer Science from Denison University (1999). His research interests include probabilistic, combinatorial, and analytic techniques for the analysis of algorithms and data structures. Since 2008, he has been the Undergraduate Chair in Statistics at Purdue, and the Associate Director for Actuarial Science. Dr. Ward is currently the Principal Investigator for the NSF grant "MCTP: Sophomore Transitions: Bridges into a Statistics Major and Big Data Research Experiences via Learning Communities" (NSF-DMS #1246818, 2013-2018). He is also an Associate Director of the Center for Science of Information (NSF-CCF #0939370, 2010-2015).
-
Ellen Gundlach
Ellen Gundlach has been teaching introductory statistics and probability classes at Purdue University as a continuing lecturer since 2002, with prior experience teaching mathematics or chemistry classes at Purdue, Ivy Tech Community College of Indiana, The Ohio State University, and Florida State University.  She is an associate editor of CAUSEweb and editor of the MERLOT Statistics Board.  Her research interests include K12 outreach activities (ASA’s first Hands-on Statistics Activity grand prize winner in 2010), online and hybrid teaching (Indiana Council for Continuing Education’s Course of the Year award in 2011), T.A. training, academic misconduct, statistical literacy, and using social media in statistics courses. She enjoys spending time with her sons Philip and Callum, playing the flute with several local groups, and supporting (and formerly skating with) the Lafayette Brawlin’ Dolls roller derby team. 
Table of Contents
I Randomness
1 Outcomes, Events, and Sample Spaces
1.1 Introduction
1.2 Complements and DeMorgans Laws
1.3 Exercises
1.3.1 Practice
1.3.2 Extensions
1.3.3 Advanced
2 Probability
2.1 Introduction
2.2 Equally-Likely Events
2.3 Complementary Probabilities; Probabilities of Subsets
2.4 Inclusion-Exclusion
2.5 More Examples of Probabilities of Events
2.6 Exercises
2.6.1 Practice
2.6.2 Extensions
2.6.3 Advanced
3 Independent Events
3.1 Introduction
3.2 Some Nice Facts About Independence
3.3 Probability of Good Occurring Before Bad
3.4 Exercises
3.4.1 Practice
3.4.2 Extensions
3.4.3 Advanced
4 Conditional Probability
4.1 Introduction
4.2 Distributive Laws
4.3 Conditional Probabilities Satisfy the Standard Probability Axioms
4.4 Exercises
4.4.1 Practice
4.4.2 Extensions
4.4.3 Advanced
5 Bayes Theorem
5.1 Introduction to Versions of Bayes Theorem
5.2 Multiplication with Conditional Probabilities
5.3 Exercises
5.3.1 Practice
5.3.2 Extensions
5.3.3 Advanced
6 Review of Randomness
6.1 Summary of Randomness
6.2 Exercises
II Discrete Random Variables
7 Discrete Versus Continuous Random Variables
7.1 Introduction
7.2 Examples
7.3 Exercises
7.3.1 Practice
7.3.2 Extensions
7.3.3 Advanced
8 Probability Mass Functions and CDFs
8.1 Introduction
8.2 Examples
8.3 Properties of the Mass and CDF
8.4 More Examples
8.5 Exercises
8.5.1 Practice
8.5.2 Extensions
8.5.3 Advanced
9 Independence and Conditioning
9.1 Joint Probability Mass Functions
9.2 Independent Random Variables
9.3 Three or More Random Variables That Are Independent
9.4 Conditional Probability Mass Functions
9.5 Exercises
9.5.1 Practice
9.5.2 Extensions
9.5.3 Advanced
10 Expected Values of Discrete Random Variables
10.1 Introduction
10.2 Examples
10.3 Exercises
10.3.1 Practice
10.3.2 Extensions
11 Expected Values of Sums of Random Variables
11.1 Introduction
11.2 Examples
11.3 Exercises
11.3.1 Practice
11.3.2 Extensions
12 Variance of Discrete Random Variables
12.1 Introduction.
12.2 Variance
12.3 Five Friendly Facts with Independence
12.4 Exercises
12.4.1 Practice
12.4.2 Extensions
12.4.3 Advanced
13 Review of Discrete Random Variables
13.1 Summary of Discrete Random Variables
13.2 Exercises
III Named Discrete Random Variables
14 Bernoulli Random Variables
14.1 Introduction
14.2 Examples
14.3 Exercises
14.3.1 Practice
14.3.2 Extensions
14.3.3 Advanced
15 Binomial Random Variables
15.1 Introduction
15.2 Examples
15.3 Exercises
15.3.1 Practice
15.3.2 Extensions
15.3.3 Advanced
16 Geometric Random Variables
6.1 Introduction
16.2 Special Features of the Geometric Distribution
16.3 The Number of Failures
16.4 Geometric Memoryless Property
16.5 Random Variables That Are Not Geometric
16.6 Exercises
16.6.1 Practice
16.6.2 Extensions
16.6.3 Advanced
17 Negative Binomial Random Variables
17.1 Introduction
17.2 Examples
17.3 Exercises
17.3.1 Practice
17.3.2 Extensions
18 Poisson Random Variables
18.1 Introduction
18.2 Sums of Independent Poisson Random Variables
18.3 Using the Poisson as an Approximation to the Binomial
18.4 Exercises
18.4.1 Practice
18.4.2 Extensions
18.4.3 Advanced
19 Hypergeometric Random Variables
19.1 Introduction
19.2 Examples
19.3 Using the Binomial as an Approximation to the Hypergeometric
19.4 Exercises
19.4.1 Practice
19.4.2 Extensions
20 Discrete Uniform Random Variables
20.1 Introduction
20.2 Examples
20.3 Exercises
20.3.1 Practice
20.3.2 Extensions
20.3.3 Advanced
21 Review of Named Discrete Random Variables
21.1 Summing up: How do you tell all these random variables apart?
21.2 Exercises
21.3 Review Problems
IV Counting
22 Introduction to Counting
22.1 Introduction
22.2 Sampling With Versus Without Replacement; With Versus Without Regard to Order
22.3 Counting: Seating Arrangements
22.4 Exercises
22.4.1 Practice
22.4.2 Extensions
22.4.3 Seating Arrangement Problems
23 Two Case Studies in Counting
23.1 Poker Hands
23.1.1 Straight Flush
23.1.2 Four Of A Kind
23.1.3 Full House
23.1.4 Flush
23.1.5 Straight
23.1.6 Three Of A Kind
23.1.7 Two Pair
23.1.8 One Pair
23.2 Yahtzee
23.2.1 Upper Section
23.2.2 Three Of A Kind
23.2.3 Four Of A Kind
23.2.4 Full House
23.2.5 Small Straight
23.2.6 Large Straight
23.2.7 Yahtzee
V Continuous Random Variables
24 Continuous Random Variables and PDFs
24.1 Introduction
24.2 Examples
24.3 Exercises
24.3.1 Practice
24.3.2 Extensions
24.3.3 Advanced
25 Joint Densities
25.1 Introduction
25.2 Examples
25.3 Exercises
25.3.1 Practice
25.3.2 Extensions
25.3.3 Advanced
26 Independent Continuous Random Variables
26.1 Introduction
26.2 Examples
26.3 Exercises
26.3.1 Practice
26.3.2 Extensions
26.3.3 Advanced
27 Conditional Distributions
27.1 Introduction
27.2 Examples
27.3 Exercises
27.3.1 Practice
27.3.2 Extensions
28 Expected Values of Continuous Random Variables
28.1 Introduction
28.2 Some Generalizations about Expected Values
28.3 Some Applied Problems with Expected Values
28.4 Exercises
28.4.1 Practice
28.4.2 Extensions
28.4.3 Advanced
29 Variance of Continuous Random Variables
29.1 Variance of a Continuous Random Variable
29.2 Expected Values of Functions of One Continuous Random Variable
29.3 Expected Values of Functions of Two Continuous Random Variables
29.4 More Friendly Facts about Continuous Random Variables
29.5 Exercises
29.5.1 Practice
29.5.2 Extensions
29.5.3 Advanced
30 Review of Continuous Random Variables
30.1 Summary of Continuous Random Variables
30.2 Exercises
VI Named Continuous Random Variables
31 Continuous Uniform Random Variables
31.1 Introduction
31.2 Examples
31.3 Linear Scaling of a Uniform Random Variable
31.4 Exercises
31.4.1 Practice
31.4.2 Extensions
31.4.3 Advanced
32 Exponential Random Variables
32.1 Introduction
32.2 Average and Variance
32.3 Properties of Exponential Random Variables
32.3.1 Complement of the CDF
32.3.2 Memoryless Property of Exponential Random Variables
32.3.3 Minimum of Independent Exponential Random Variables
32.3.4 Poisson Process
32.3.5 Moments of an Exponential Random Variable (Optional)
32.4 Exercises .
32.4.1 Practice
32.4.2 Extensions
32.4.3 Advanced
33 Gamma Random Variables
33.1 Introduction
33.2 Examples
33.3 Exercises
33.3.1 Practice
33.3.2 Extensions
33.3.3 Advanced
34 Beta Random Variables
34.1 Introduction
34.2 Examples
34.3 Exercises
34.3.1 Practice
34.3.2 Extensions
35 Normal Random Variables
35.1 Introduction
35.2 Using the Normal Distribution: Scaling and Transforming to Standard Normal
35.3 \Backwards" Normal Problems
35.4 Summary: How to Distinguish a \Forward" Versus \Backwards" Normal Problem?
35.5 Exercises
35.5.1 Practice
35.5.2 Extensions
35.5.3 Advanced
36 Sums of Independent Normal Random Variables
36.1 The Sum of Independent Normal Random Variables is Normally Distributed
36.2 Why the Sum of Independent Normals is Normal Too (Optional)
36.3 Exercises
36.3.1 Practice
36.3.2 Extensions
36.3.3 Advanced
37 Central Limit Theorem
37.1 Introduction
37.2 Laws of Large Numbers
37.3 Central Limit Theorem
37.4 Applications of the Central Limit Theorem to Sums of Continuous Random Variables
37.5 Applications of the Central Limit Theorem to Sums of Discrete Random Variables
37.6 Normal Approximations to Binomial Random Variables
37.7 Normal Approximations to Poisson Random Variables
37.8 Exercises
37.8.1 Practice
37.8.2 Extensions
38 Review of Named Continuous Random Variables
38.1 Summing up: How do you tell all these random variables apart?
38.2 Exercises
VII Additional Topics
39 Variance of Sums; Covariance; Correlation
39.1 Introduction
39.2 Motivation for Covariance
39.3 Properties of the Covariance
39.4 Examples of Covariance
39.5 Linearity of the Covariance
39.6 Correlation
39.7 Exercises
39.7.1 Practice
39.7.2 Extensions
39.7.3 Advanced
40 Conditional Expectation
40.1 Introduction
40.2 Examples
40.3 Exercises
40.3.1 Practice
40.3.2 Extensions
40.3.3 Advanced.
41 Markov and Chebyshev Inequalities
41.1 Introduction
41.2 Markov Inequality
41.3 Chebyshev Inequality
41.4 Exercises
41.4.1 Practice
41.4.2 Extensions
42 Order Statistics
42.1 Introduction
42.2 Examples
42.3 Joint Density and Joint CDF of Order Statistics
42.4 Exercises
42.4.1 Practice
42.4.2 Extensions
43 Moment Generating Functions
43.1 A Brief Introduction to Generating Functions
43.2 Moment Generating Functions
43.3 Moment Generating Functions of Discrete Random Variables
43.4 Moment Generating Functions of Continuous Random Variables
43.5 Appendix: Building a Generating Function
43.6 Exercises
44 Transformations of One or Two Random Variables
44.1 Distribution of a Function of One Continuous Random Variable
44.2 Joint Density of Two Random Variables That Are Functions of Another Pair of Random Variables
44.3 Exercises
44.3.1 Practice
44.3.2 Extensions
44.3.3 Advanced
45 Review Questions for All Chapters
Product Updates
Unlike most probability textbooks, which are only truly accessible to mathematically-oriented students, Ward and Gundlach’s Introduction to Probability reaches out to a much wider introductory-level audience. Its conversational style, highly visual approach, practical examples, and step-by-step problem solving procedures help all kinds of students understand the basics of probability theory and its broad applications. The book was extensively class-tested through its preliminary edition, to make it even more effective at building confidence in students who have viable problem-solving potential but are not fully comfortable in the culture of mathematics.
Looking for instructor resources like Test Banks, Lecture Slides, and Clicker Questions? Request access to Achieve to explore the full suite of instructor resources.
Instructor Resources
Instructor Resources
Download Resources
You need to sign in to unlock your resources.
You've selected:
Click the E-mail Download Link button and we'll send you an e-mail at with links to download your instructor resources. Please note there may be a delay in delivering your e-mail depending on the size of the files.
Warning! These materials are owned by Macmillan Learning or its licensors and are protected by copyright laws in the United States and other jurisdictions. Such materials may include a digital watermark that is linked to your name and email address in your Macmillan Learning account to identify the source of any materials used in an unauthorised way and prevent online piracy. These materials are being provided solely for instructional use by instructors who have adopted Macmillan Learning’s accompanying textbooks or online products for use by students in their courses. These materials may not be copied, distributed, sold, shared, posted online, or used, in print or electronic format, except in the limited circumstances set forth in the Macmillan Learning Terms of Use and any other reproduction or distribution is illegal. These materials may not be made publicly available under any circumstances. All other rights reserved. For more information about the use of your personal data including for the purposes of anti-piracy enforcement, please refer to Macmillan Learning's.Privacy Notice
Thank you!
Your download request has been received and your download link will be sent to .
Please note you could wait up to 30 to 60 minutes to receive your download e-mail depending on the number and size of the files. We appreciate your patience while we process your request.
Check your inbox, trash, and spam folders for an e-mail from InstructorResources@macmillan.com.
If you do not receive your e-mail, please visit macmillanlearning.com/support.
FAQs
-
-
Are you a campus bookstore looking for ordering information?
MPS Order Search Tool (MOST) is a web-based purchase order tracking program that allows customers to view and track their purchases. No registration or special codes needed! Just enter your BILL-TO ACCT # and your ZIP CODE to track orders.
Canadian Stores: Please use only the first five digits/letters in your zip code on MOST.
Visit MOST, our online ordering system for booksellers: https://tracking.mpsvirginia.com/Login.aspx
Learn more about our Bookstore programs here: https://www.macmillanlearning.com/college/us/contact-us/booksellers
-
-
-
Our courses currently integrate with Canvas, Blackboard (Learn and Ultra), Brightspace, D2L, and Moodle. Click on the support documentation below to find out more details about the integration with each LMS.
Integrate Macmillan courses with Blackboard
Integrate Macmillan courses with Canvas
-
-
-
If you’re a verified instructor, you can request a free sample of our courseware, e-book, or print textbook to consider for use in your courses. Only registered and verified instructors can receive free print and digital samples, and they should not be sold to bookstores or book resellers. If you don't yet have an existing account with Macmillan Learning, it can take up to two business days to verify your status as an instructor. You can request a free sample from the right side of this product page by clicking on the "Request Instructor Sample" button or by contacting your rep. Learn more.
-
-
-
Sometimes also referred to as a spiral-bound or binder-ready textbook, loose-leaf textbooks are available to purchase. This three-hole punched, unbound version of the book costs less than a hardcover or paperback book.
-
-
-
Achieve (full course) includes our complete e-book, as well as online quizzing tools, multimedia assets, and iClicker active classroom manager.
Most Achieve Essentials courses do not include our e-books and adaptive quizzing.
Visit our comparison table for details: https://www.macmillanlearning.com/college/us/digital/achieve/compare
-
-
-
Achieve (full course) includes our complete e-book, as well as online quizzing tools, multimedia assets, and iClicker active classroom manager.
Achieve Read & Practice only includes our e-book and adaptive quizzing, and does not include instructor resources and assignable assessments. Read & Practice does integrate with LMS.
Visit our comparison table for details: https://www.macmillanlearning.com/college/us/digital/achieve/compare
-
-
-
We can help! Contact your representative to discuss your specific needs for your course. If our off-the-shelf course materials don’t quite hit the mark, we also offer custom solutions made to fit your needs.
-
ISBN:9781319049393
Take notes, add highlights, and download our mobile-friendly e-books.
ISBN:9780716771098
Read and study old-school with our bound texts.
ISBN:9781319060893
Take notes, add highlights, and download our mobile-friendly e-books.
Introduction to Probability
Unlike most probability textbooks, which are only truly accessible to mathematically-oriented students, Ward and Gundlach’s Introduction to Probability reaches out to a much wider introductory-level audience. Its conversational style, highly visual approach, practical examples, and step-by-step problem solving procedures help all kinds of students understand the basics of probability theory and its broad applications. The book was extensively class-tested through its preliminary edition, to make it even more effective at building confidence in students who have viable problem-solving potential but are not fully comfortable in the culture of mathematics.
Select a demo to view:
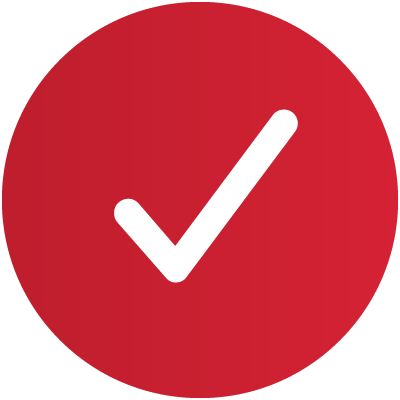